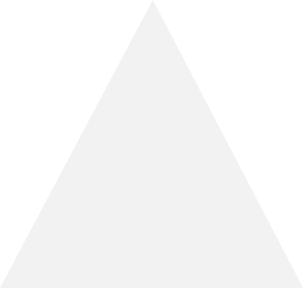
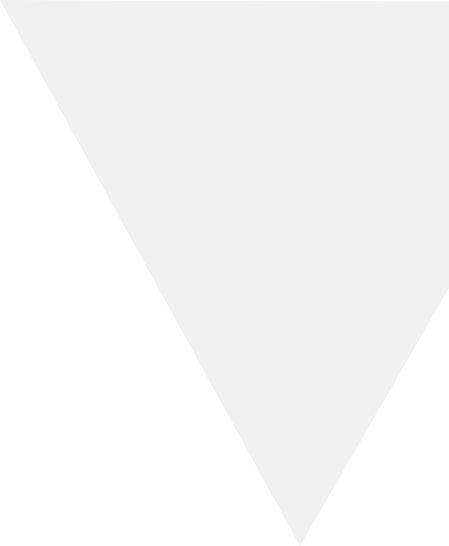
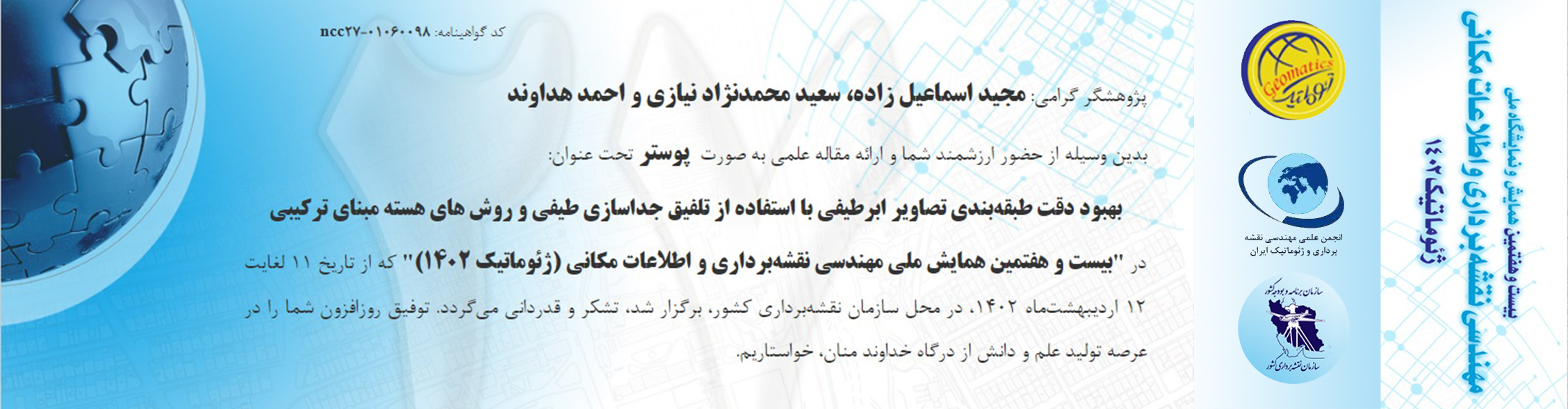
The authors : Majid Esmaeilzadeh, Saeed Mohammadnejad Niyazi, Ahmad Hadavand
Place of publication : 27th Conference on Surveying & Spatial Information (Geomatics 1402)
Place of publication : 2023
Abstract:
Hyperspectral sensors measure the scattered electromagnetic energy in their instantaneous field of view across hundreds or thousands of spectral bands, with higher spectral resolution compared to multispectral sensors. This appropriate spectral resolution enables the identification of materials through spectral analysis. However, due to the low spatial resolution of hyperspectral sensors, mixing of materials, and scattering of signals in different directions, the spectrum recorded by hyperspectral sensors is a mixture of the spectra of different materials present in the imaging scene. Therefore, accurate estimation requires spectral unmixing. The primary objective of this paper is to improve the classification accuracy of hyperspectral images. To achieve this, two classified maps are integrated; one map is created using spectral unmixing methods, and the other through hyperspectral image classification methods. The employed methods utilize both spectral and spatial information. After preparing the two classified maps, they are combined using one of the decision-level fusion methods. The proposed method has been implemented on three reference hyperspectral datasets. In all these datasets, improvements in performance and classification accuracy are clearly observable, with the highest accuracies achieved ranging from 96% to 99.5%.