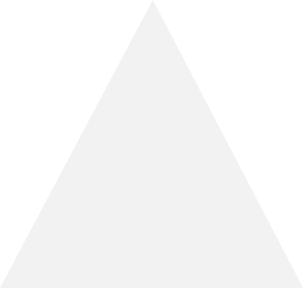
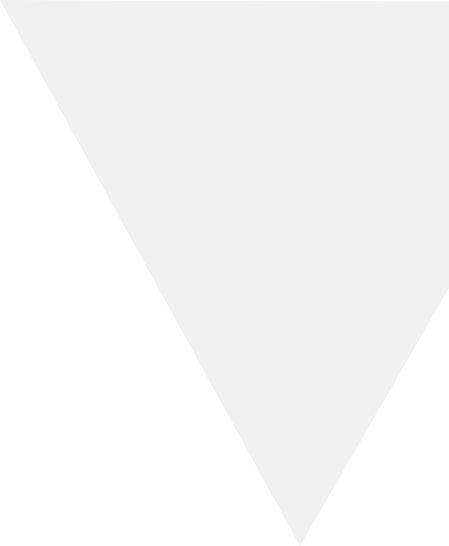
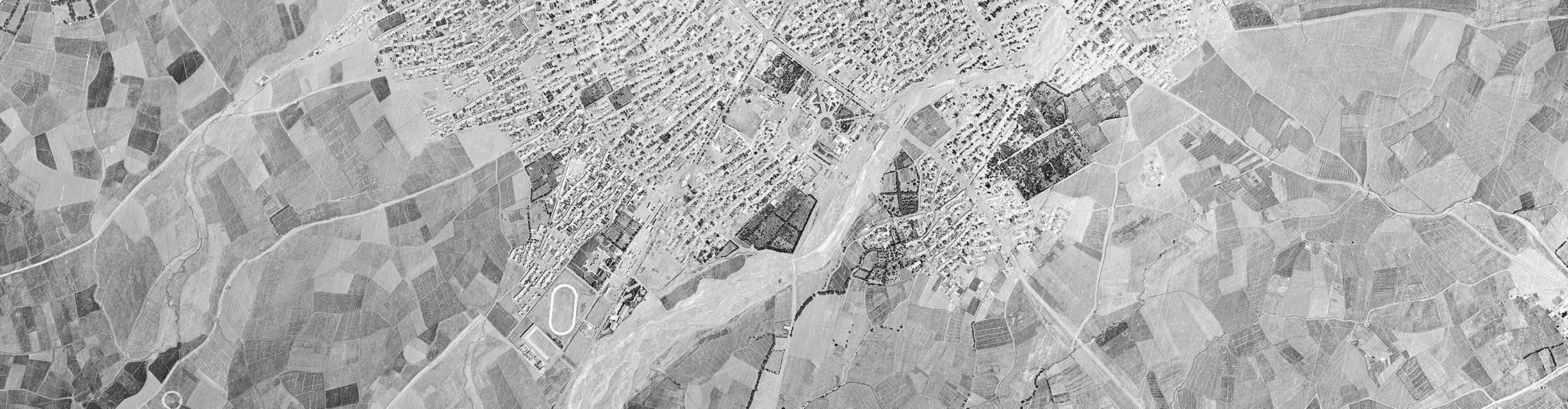
The authors : صالح آقایاری، احمد هداوند، سعید محمدنژاد نیازی، محمد امید علی نظری
Place of publication : ISPRS Annals of the Photogrammetry, Remote Sensing and Spatial Information Sciences, Volume X-4/W1-2022, GeoSpatial Conference 2022
Place of publication : 2022
:ABSTRACT
Buildings are one of the key components in change detection, urban planning, and monitoring. The automatic extraction of the building from high-resolution aerial imagery is still challenging due to the variations in their shapes, structures, textures, and colours. Recently, the convolutional neural networks (CNN) show a significant improvement in object detection and extraction that surpasses other methods. To extract building, in this paper two segmentation architectures, the UNet and the Inception ResNet UNet are
implemented and then tested on the Inria aerial image datasets. The Inception ResNet UNet utilizes the Inception architecture and residual blocks. This makes the model wide and deep, though there are a few differences between numbers of UNet and Inception ResNet UNet parameters. The analyses show that UNet has a high rate of metrics in the training progress. However, on the unseen dataset, Inception ResNet UNet extracts buildings more accurately (97.95% accuracy and 0.96 in the dice metric) in comparison with UNet (94.30% accuracy and 0.55 in the dice metric)
KEY WORDS: Large-scale monitoring, Building detection, Image segmentation, Residual blocks, Skip connection